A match made in heaven: Tinder and Statistics — Insights from an unique Dataset of swiping
Motivation
Tinder is a huge phenomenon in the online dating world. Because of its massive user base it potentially offers lots of data that is exciting to analyze. A general overview on Tinder can be found in this article which mainly looks at business key figures and surveys of users:
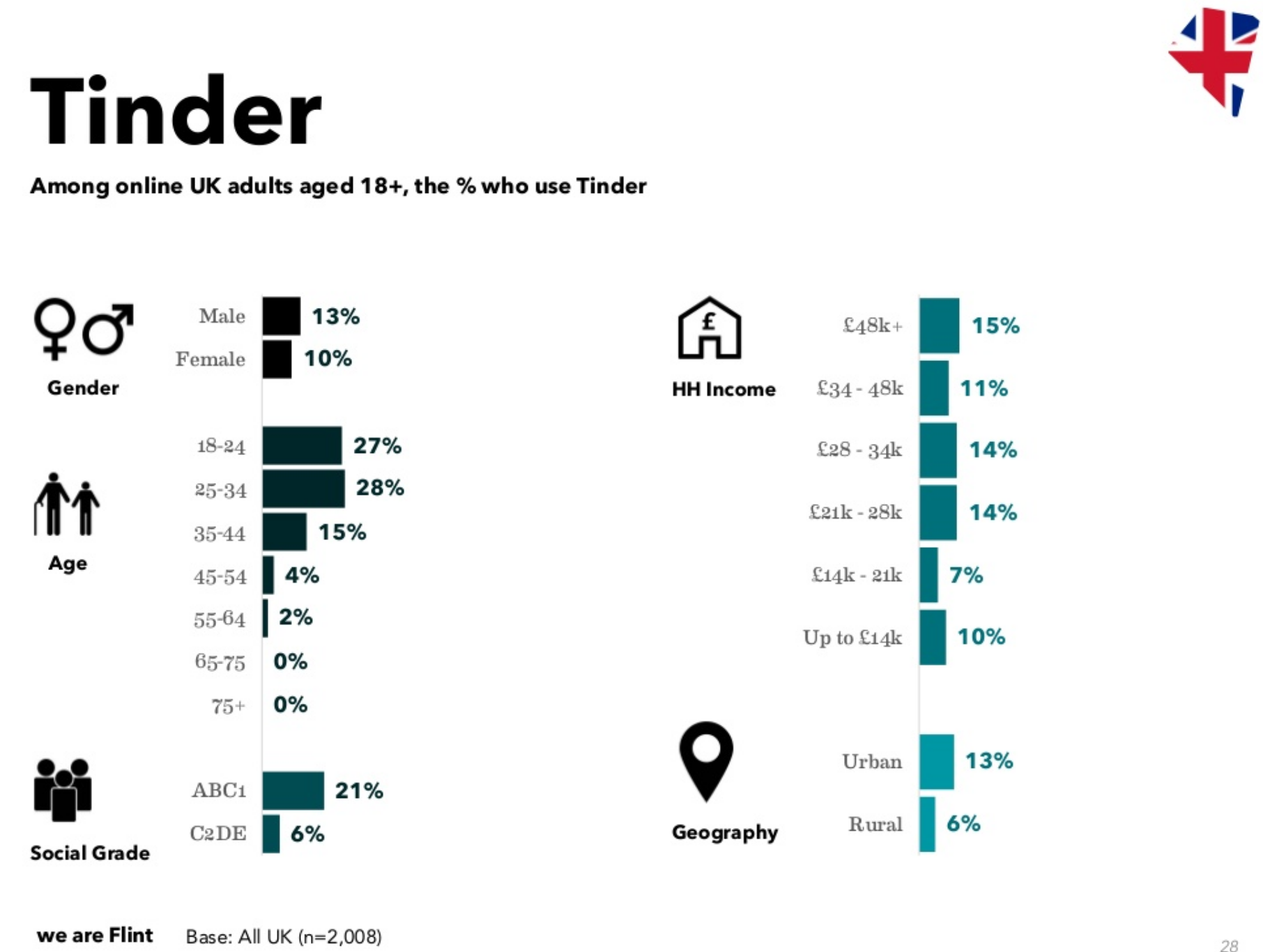
However, there are only sparse resources looking at Tinder app data on a user level. One reason for that being that data is not easy to gather. One approach is to ask Tinder for your own data. This process was used in this inspiring analysis which focuses on matching rates and messaging between users. Another way is to create profiles and automatically collect data on your own by using the undocumented Tinder API. This method was used in a paper which is summarized neatly in this blogpost. The paper’s focus also was the study of matching and messaging behavior of users. Lastly, this post summarizes finding from the biographies of male and female Tinder profiles from Sydney.
In the following, we will complement and expand previous analyses on Tinder data. Using an unique, extensive dataset we will apply descriptive statistics, natural language processing and visualizations in order to uncover patterns on Tinder. In this first analysis we will focus on insights from profiles we observe during swiping as a male. What is more, we observe female profiles from swiping as a heterosexual as well as male profiles from swiping as a homosexual. In this follow up post we then look at novel findings from a field experiment on Tinder. The results will reveal new insights regarding liking behavior and patterns in matching and messaging of users.
Data collection
The dataset was gathered using bots making use of the unofficial Tinder API. The bots used two almost identical male profiles aged 29 to swipe in Germany. There were two consecutive phases of swiping, each over the course of four weeks. After each week, the location was set to the city center of one of the following cities: Berlin, Frankfurt, Hamburg and Munich. The distance filter was set to 16km and age filter to 20-40. The search preference was set to women for the heterosexual and respectively to men for the homosexual treatment. Each bot encountered about 300 profiles per day. The profile data was returned in JSON
format in batches of 10-30 profiles per response.
Unfortunately, I won’t be able to share the dataset because doing so is in a gray area. Check out this post to learn about the many legal issues that come with such datasets.
Setting up things
In the following, I will share my data analysis of the dataset using a Jupyter Notebook. So, let’s get started by first importing the packages we will use and setting some options:
# coding: utf-8
import pandas as pd
import numpy as np
import nltk
import textblob
import datetime
from wordcloud import WordCloud
from PIL import Image
from IPython.display import Markdown as md
from pandas.io.json import json_normalize
import hvplot.pandas
#from bokeh.io import output_notebook
#output_notebook()
pd.set_option('display.max_columns', 100)
from IPython.core.interactiveshell import InteractiveShell
InteractiveShell.ast_node_interactivity = "all"
import holoviews as hv
hv.extension('bokeh')
Most packages are the basic stack for any data analysis. In addition, we will use the wonderful hvplot library for visualization. Until now I was overwhelmed by the vast choice of visualization libraries in Python (here is a great read on that). This ends with hvplot which comes out of the PyViz initiative. It is a high-level library with a concise syntax that produces not only aesthetic but also interactive plots. Among others, it smoothly works on pandas
DataFrames.
With json_normalize
we can easily create flat tables from deeply nested json files. The Natural Language Toolkit (nltk) and Textblob will be used to deal with language and text. And finally wordcloud does what it says.
We have already converted the json
data to csv so we go ahead and read it in:
profiles = pd.read_csv("./data/interim/recommendations_1-4.csv")
profiles.head()
_id | bio | birth_date | birth_date_info | common_friend_count | common_friends | common_like_count | common_likes | connection_count | content_hash | distance_mi | gender | group_matched | jobs | name | photos | ping_time | s_number | schools | teasers | teaser.string | teaser.type | instagram.completed_initial_fetch | instagram.last_fetch_time | instagram.media_count | instagram.photos | instagram.profile_picture | instagram.username | spotify_theme_track.album.id | spotify_theme_track.album.images | spotify_theme_track.album.name | spotify_theme_track.artists | spotify_theme_track.id | spotify_theme_track.name | spotify_theme_track.preview_url | spotify_theme_track.uri | is_traveling | hide_age | hide_distance | show_gender_on_profile | scrape_time | city | bot | spotify_top_artists | custom_gender | is_super_like | |
---|---|---|---|---|---|---|---|---|---|---|---|---|---|---|---|---|---|---|---|---|---|---|---|---|---|---|---|---|---|---|---|---|---|---|---|---|---|---|---|---|---|---|---|---|---|---|
0 | 5bc5ba38759ed8e42565d9b5 | NaN | 1983-07-21T08:26:56.474Z | fuzzy birthdate active, not displaying real bi... | 0 | [] | 0 | [] | 0 | mvjimkIGbcP3IbOfeDIm3fDXu2QUwUnNup5cGliVs9Oum5 | 2 | 0 | False | [{'title': {'name': 'Syndikus'}}] | Simon | [{'crop_info': {'algo': {'height_pct': 0.63790... | 2014-12-09T00:00:00.000Z | 627765497 | [{'name': 'Ludwig-Maximilians-Universität Münc... | [{'string': 'Syndikus', 'type': 'job'}, {'stri... | Syndikus | job | NaN | NaN | NaN | NaN | NaN | NaN | NaN | NaN | NaN | NaN | NaN | NaN | NaN | NaN | NaN | NaN | NaN | NaN | 2019-07-18 08:26:56 | munich | 3 | NaN | NaN | NaN |
1 | 5d00ff970e38f71500ca94b1 | NaN | 1982-07-21T08:26:56.474Z | fuzzy birthdate active, not displaying real bi... | 0 | [] | 0 | [] | 0 | Dppi6zfbls2DIxMFlcQaH1jH9piRqIZRf2AspGcG2igzIG | 3 | 0 | False | [] | Ben | [{'crop_info': {'processed_by_bullseye': True,... | 2014-12-09T00:00:00.000Z | 769017892 | [{'name': 'University of Melbourne'}] | [{'string': 'University of Melbourne', 'type':... | University of Melbourne | school | NaN | NaN | NaN | NaN | NaN | NaN | NaN | NaN | NaN | NaN | NaN | NaN | NaN | NaN | NaN | NaN | NaN | NaN | 2019-07-18 08:26:56 | munich | 3 | NaN | NaN | NaN |
2 | 5c3b8ae5e9b668dc71c9d3b9 | NaN | 1996-07-21T08:26:56.474Z | fuzzy birthdate active, not displaying real bi... | 0 | [] | 0 | [] | 0 | Q6JFJlt4Ah0gTeohz6UxOUXJFgXTgPhRsNnfkJU2dhvJhpP | 1 | 0 | False | [{'title': {'name': 'Gesundheits und Krankenpf... | Michi | [{'crop_info': {'algo': {'height_pct': 0.33038... | 2014-12-09T00:00:00.000Z | 672409017 | [] | [{'string': 'Gesundheits und Krankenpfleger', ... | Gesundheits und Krankenpfleger | job | True | 2019-07-18T07:33:28.737Z | 134.0 | [{'image': 'https://scontent.cdninstagram.com/... | https://scontent.cdninstagram.com/vp/cab9e2467... | Tinder | NaN | NaN | NaN | NaN | NaN | NaN | NaN | NaN | NaN | NaN | NaN | NaN | 2019-07-18 08:26:56 | munich | 3 | NaN | NaN | NaN |
3 | 5cf55b62df730c1500d79634 | Immer auf der Suche nach neuen, interessanten ... | 1993-07-21T08:26:56.474Z | fuzzy birthdate active, not displaying real bi... | 0 | [] | 0 | [] | 0 | Get8rcLziGxHnf1XtNAUbuOgFbinYT64un6I9efAH20 | 1 | 0 | False | [{'title': {'name': 'Student'}}] | Max | [{'crop_info': {'algo': {'height_pct': 0.38030... | 2014-12-09T00:00:00.000Z | 764275080 | [{'name': 'TUM'}] | [{'string': 'Student', 'type': 'job'}, {'strin... | Student | job | NaN | NaN | NaN | NaN | NaN | NaN | 1OydCrx4m7fguwcX4stR9z | [{'height': 640, 'url': 'https://i.scdn.co/ima... | Trading Snakeoil for Wolftickets | [{'id': '5oRnbmgqvvq7fVlgk4vcEa', 'name': 'Gar... | 3JOVTQ5h8HGFnDdp4VT3MP | Mad World (Feat. Michael Andrews) | https://p.scdn.co/mp3-preview/e2425aabb084ac6d... | spotify:track:3JOVTQ5h8HGFnDdp4VT3MP | NaN | NaN | NaN | NaN | 2019-07-18 08:26:56 | munich | 3 | NaN | NaN | NaN |
4 | 5c364540d8fca5055e7738b5 | Auf der Suche nach allem und nichts. \nFinds r... | 1991-07-21T08:26:56.474Z | fuzzy birthdate active, not displaying real bi... | 0 | [] | 0 | [] | 0 | ob5TGwtLXtPOTbLip5uxDIkpce5f18ID2UYoCvNseViqPC6j | 3 | 0 | False | [{'company': {'name': 'München, Bayern'}, 'tit... | Mario | [{'crop_info': {'algo': {'height_pct': 0.27501... | 2014-12-09T00:00:00.000Z | 686984950 | [] | [{'string': 'Steuerfachangestellter at München... | Steuerfachangestellter at München, Bayern | jobPosition | NaN | NaN | NaN | NaN | NaN | NaN | NaN | NaN | NaN | NaN | NaN | NaN | NaN | NaN | False | NaN | NaN | NaN | 2019-07-18 08:26:56 | munich | 3 | NaN | NaN | NaN |
profiles = profiles[profiles['city']!='ddorf']
Basically, we have all the info that makes up a tinder profile. Moreover, we have some additional data which might not be obivous when using the app. For example, the hide_age
and hide_distance
variables indicate whether the person has a premium account (those are premium features). Usually, they are NaN
but for paying users they are either True
or False
. Paying users can either have a Tinder Plus or Tinder Gold subscription. In addition, teaser.string
and teaser.type
are empty for most profiles. In some cases they are not. I would guess that this indicates profiles showing up in the top picks part of the app.
Some general figures
Let’s see how many profiles there are in the data. Also, we’ll check how many profile we’ve encountered multiple times while swiping. For that, we’ll look at the number of duplicates. Moreover, let’s see what fraction of people are paying premium users:
# treatment 1 = hetero = women treatment 2 = homo = man
profiles['homo'] = np.where(profiles['bot'] <= 2, 0, 1)
profiles['treatment'] = np.where(profiles['bot'] <= 2, 'hetero', 'homo')
num_profiles = profiles.groupby(['homo', 'bot'])['_id'].count()
In total we have observed 25700 profiles during swiping. Out of those, 16673 in treatment one (straight) and 9027 in treatment two (gay).
# Count duplicated
num_dups_bot = profiles[profiles.duplicated(subset=['_id', 'bot'])]\
.groupby('bot')['_id'].agg('nunique')
num_dups_treat = profiles[profiles.duplicated(subset=['_id', 'homo'])]\
.groupby('homo')['_id'].agg('nunique')
share_dups_bot = num_dups_bot / num_profiles * 100
share_dups_treat = num_dups_treat / num_profiles.groupby('homo').sum() * 100
share_premium = profiles.groupby('homo')['hide_age'].count()\
/ profiles.groupby('homo')['_id'].count() * 100
On average, a profile is only encountered repeatedly in 0.6% of the cases per bot. In conclusion, if you don’t swipe excessively in the same area it is very improbable to see a person twice. In 12.3% (women), respectively 16.1% (men) of the cases a profile was suggested to both our bots. Taking into account the number of profiles observed in total, this shows that the total user base must be huge for the cities we swiped in. Also, the gay user base must be significantly lower. Our next interesting finding is the share of premium users. We find 8.1% for women and 20.9% for gay men. Thus, men are much more willing to spend money in exchange for better chances in the matching game. On top of that, Tinder is quite good at acquiring paying users in general.
I’m old enough to be …
Next, we drop the duplicates and start looking at the data in more depth. We begin by calculating the age of the profiles and visualizing its distribution:
profiles = profiles.drop_duplicates(['_id'], keep='last').reset_index(drop=True)
# Deal with Time and calculate age - original Analysis done on 2019-07-01
NOW = pd.to_datetime(datetime.datetime.utcnow().strftime("%Y-%m-%d %H:%M:%S %z"),
utc=True)
profiles['birth_date'] = pd.to_datetime(profiles['birth_date'])
profiles['age'] = profiles['birth_date']\
.map(lambda x: np.floor((NOW - x) / np.timedelta64(1,'Y')))
# Calc rel. frequencies and overlay two bar charts
homo = profiles[profiles['homo']==1]
hetero = profiles[profiles['homo']==0]
age_plot = pd.crosstab(homo['age'], homo['city'], normalize='columns')\
.apply(lambda x: x * 100).stack()\
.hvplot.bar(label="homo")\
.groupby("city").opts(alpha=0.3, muted_alpha=0.1) *\
pd.crosstab(hetero['age'], hetero['city'], normalize='columns')\
.apply(lambda x: x * 100).stack()\
.hvplot.bar(label="hetero")\
.groupby("city").opts(alpha=0.3, muted_alpha=0.1)
age_plot.opts(width=500, height=350, ylabel='freq.', yformatter='%.0f%%',
title="Age of profiles observed")
The most obvious finding is the stark difference between treatments. In both cases, the distribution of ages we encounter comes close to a normal distribution with a mean of 28. However, swiping as a gay male we see a much more even distribution than swiping as a straight male were ages are more concentrated around 27 to 30. For the gay profile the encountered ages are pretty similar between cities. In contrast, for the straight profile we see some differences. Ages in Berlin are more concentrated around the mean while in Frankfurt they are more evenly distributed. However, this is not a mere representation of the respective tinder user base. It is biased because there is an age-filter which works both ways: Tinder will only display profiles that are not only within your defined limits but for which you are withnin their respective age limits as well. Apparently, women prefer to swipe on men similar in age. Still, the right skew of the distribution indicates that older men are prefered to younger ones. Gay men have a wider age range in their filters. Also, the share of older men looking for younger men is much higher then it is for women.
Say my name, say my name….
Tinder shows the first name of its users. Using that, let’s look at the most popular names that we came across:
from collections import Counter
# Table with topN profile names
wordcount_homo = Counter(homo['name']).most_common(10)
wordcount_hetero = Counter(hetero['name']).most_common(10)
df_homo = pd.DataFrame(wordcount_homo, columns=['name', 'count'])
df_hetero = pd.DataFrame(wordcount_hetero, columns=['name', 'count'])
top10 = df_homo.merge(df_hetero, left_index=True,
right_index=True, suffixes=('_homo', '_hetero'))
top10.hvplot.table(width=330)
As expected, very common German names are overrepresented in our sample. Daniel and Julia definitely take home the trophy for the most common names we observe.
It’s all about the pictures
Your looks contribute vastly to how attractive you are perceived especially lacking other information. That’s more true on Tinder than anywhere else and the reason why success here is all about your pictures. This is why you can upload up to ten profile pictures. Profiles with only one picture often seem fishy. But ten pictures? Really? That might come off as desperate. So, how many is too many? Let us investigate how females decide here:
import re
# Count number of photos
profiles['num_profile_photos'] = profiles['photos']\
.map(lambda x:
len(re.findall("fileName", x)))
profiles.groupby('treatment')['num_profile_photos'].describe()
count | mean | std | min | 25% | 50% | 75% | max | |
---|---|---|---|---|---|---|---|---|
treatment | ||||||||
hetero | 14590.0 | 4.644825 | 2.227076 | 1.0 | 3.0 | 4.0 | 6.0 | 10.0 |
homo | 7558.0 | 5.249802 | 2.260475 | 1.0 | 4.0 | 5.0 | 7.0 | 10.0 |
# Calc rel. freq. of photos and show grouped bar chart by treat.
photos = profiles.groupby(['num_profile_photos', 'treatment'])['_id'].count()\
.unstack().transform(lambda x: x / x.sum() * 100)
# workaround for bug in holoviews regarding x-axis ordering
photos.index = photos.index.map(str)
photos.index = photos.index.map(lambda x: '0' + x if len(x) < 2 else x)
photos.hvplot.bar(xlabel='pictures', ylabel='freq.', width=700, height=350,
rot=45, title='Number of profile pictures',
yformatter="%f%%")
First, we compare the inter-quantile-range (IQR) of the number of profile pictures between treatments. We see that women mostly use three to six pictures while gay men use four to seven. The difference is largets at both ends of the distribution. In both cases, the abrupt drop between nine and ten pictures is striking. It might be that people don’t want to come across as deperate or vain by exhausting the limit. However, quite a few people settle for a single picture. Particulary women. Does this have anything to do with age? Let’s check:
profiles.groupby('treatment')[['age', 'num_profile_photos']].corr()
age | num_profile_photos | ||
---|---|---|---|
treatment | |||
hetero | age | 1.000000 | 0.057304 |
num_profile_photos | 0.057304 | 1.000000 | |
homo | age | 1.000000 | 0.082492 |
num_profile_photos | 0.082492 | 1.000000 |
A basic correlation between age
and num_profile_photos
shows no link. However, this is a perfect lesson on why one should be wary of correlations. We can only conclude that there is no linear link between those variables. But what about non-linear dependencies? A truly interesting pattern emerges when we depict both variables in a scatter plot:
profiles.groupby(['age', 'treatment'])['num_profile_photos']\
.mean().hvplot.scatter(by='treatment')\
.opts(width=600, height=350,
title='Average number of profile pictures by age')
For the homo treatment we find a linear increase of the average number of profiles pictures from the age 21 to 30. Thereafter, it stays constant. For the hetero treatment we get an almost perfect inversed U-shape: you can clearly see how the positive association peaks around 31 and then drops quickly. For me, this pattern is somewhat surprising. I would have expected younger people to be more involved in their profiles thus sharing more pictures.
We can further investigate these patterns by looking at Instagram (IG). As users can link their IG account with Tinder to share even more pictures we have data on that as well. We can investigate the frequency of linked IG accounts and also the number of IG pictures by age. Here, again, I expect younger people to be more willing to share. Let’s see if my hunch is right this time:
profiles['instagram.media_count'] = profiles['instagram.media_count'].fillna(0)
profiles.groupby('treatment')['instagram.media_count'].describe()
count | mean | std | min | 25% | 50% | 75% | max | |
---|---|---|---|---|---|---|---|---|
treatment | ||||||||
hetero | 14590.0 | 25.322138 | 112.381127 | 0.0 | 0.0 | 0.0 | 0.0 | 2545.0 |
homo | 7558.0 | 74.698465 | 258.661286 | 0.0 | 0.0 | 0.0 | 30.0 | 8950.0 |
IG_max = profiles.groupby('treatment')['instagram.media_count'].max()
IG_share = profiles[profiles['instagram.media_count'] > 0]\
.groupby('treatment')['_id'].count()\
/ profiles.groupby('treatment')['instagram.media_count'].count() * 100
IG_excessive = profiles[profiles['instagram.media_count'] > 1000]\
.groupby('treatment')['_id'].count()
profiles[['treatment','instagram.media_count']].hvplot\
.hist(by='treatment', bins=200, xlim=(0, 2000),
alpha=0.3, muted_alpha=0.1,
title="Histogram of number of instagram pictures",
xlabel="IG pictures", ylabel="count",
width=600, height=350)
Surprisingly, only 12% of women link their IG account. In contrast, for gay men that share is 29%. Amongst those who do there are 32 females and 105 males with more than 1000 pictures on IG. The top numbers we observe are 2545 respectively 8950. That seems a little bit excessive… Fortunately, this exaggerated use of IG can only be observed in less than 1% of our sample. So most people we encounter seem to still have a live besides that. The general picture here is in line with the pattern observed earlier: gay men share significantly more pictures than women. In terms of correlation with age: there is none. So again, I have been proven wrong with my intuition.
A picture is worth a thousand words. But still …
Obviously pictures are the most important feature of a tinder profile. Also, age plays an important role because of the age filter. But there is one more piece to the puzzle: the biography text (bio). While some don’t use it at all some seem to be very wary about it. The text can be used to describe oneself, to state expectations or in some cases just to be funny:
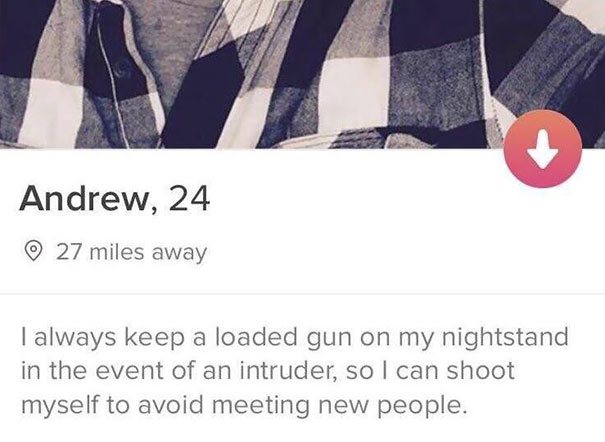
For that, you have a limit of 500 characters. Let’s see what we can learn from the bios:
# Calc some stats on number of chars
profiles['bio_num_chars'] = profiles['bio'].str.len()
profiles.groupby('treatment')['bio_num_chars'].describe()
count | mean | std | min | 25% | 50% | 75% | max | |
---|---|---|---|---|---|---|---|---|
treatment | ||||||||
hetero | 8577.0 | 100.647196 | 114.607153 | 1.0 | 21.0 | 55.0 | 136.0 | 500.0 |
homo | 5461.0 | 118.364036 | 115.112089 | 1.0 | 33.0 | 79.0 | 167.0 | 500.0 |
bio_chars_mean = profiles.groupby('treatment')['bio_num_chars'].mean()
bio_text_yes = profiles[profiles['bio_num_chars'] > 0]\
.groupby('treatment')['_id'].count()
bio_text_100 = profiles[profiles['bio_num_chars'] > 100]\
.groupby('treatment')['_id'].count()
bio_text_share_no = (1- (bio_text_yes /\
profiles.groupby('treatment')['_id'].count())) * 100
bio_text_share_100 = (bio_text_100 /\
profiles.groupby('treatment')['_id'].count()) * 100
In 41% (28% ) of the cases females (gay males) didn’t use the biography at all. The average female (male) observed has around 101 (118) characters in her (his) bio. And only 19.6% (30.2%) seem to put some emphasis on the text by using more than 100 characters. These findings suggest that text only plays a minor role on Tinder profiles and more so for women. However, while naturally pictures are essential text might have a more subtle part. For example, emojis (or hashtags) are often used to describe one’s preferences in a very character efficient way. This strategy is in line with communication in other online channels like Twitter or WhatsApp. Hence, we’ll take a look at emoijs and hashtags later on.
What can we learn from the content of biography texts? To answer this, we will need to dive into Natural Language Processing (NLP). For this, we will use the nltk and Textblob libraries. Some informative introductions on the topic can be found here and here. They describe all methods applied here. We start by looking at the most common words. For that, we need to get rid of very common words (stopwords). Following, we can look at the number of occurrences of the remaining, used words:
# Filter out English and German stopwords
from textblob import TextBlob
from nltk.corpus import stopwords
profiles['bio'] = profiles['bio'].fillna('').str.lower()
stop = stopwords.words('english')
stop.extend(stopwords.words('german'))
stop.extend(("’", "’", "‘", "“", "„"))
def remove_stop(x):
#remove stop words from sentence and return str
return ' '.join([word for word in TextBlob(x).words if word.lower() not in stop])
profiles['bio_clean'] = profiles['bio'].map(lambda x:remove_stop(x))
# Single String with all texts
bio_text_homo = profiles.loc[profiles['homo'] == 1, 'bio_clean'].tolist()
bio_text_hetero = profiles.loc[profiles['homo'] == 0, 'bio_clean'].tolist()
bio_text_homo = ' '.join(bio_text_homo)
bio_text_hetero = ' '.join(bio_text_hetero)
# Count word occurences, convert to df and show table
wordcount_homo = Counter(TextBlob(bio_text_homo).words).most_common(50)
wordcount_hetero = Counter(TextBlob(bio_text_hetero).words).most_common(50)
top50_homo = pd.DataFrame(wordcount_homo, columns=['word', 'count'])\
.sort_values('count', ascending=False)
top50_hetero = pd.DataFrame(wordcount_hetero, columns=['word', 'count'])\
.sort_values('count', ascending=False)
top50 = top50_homo.merge(top50_hetero, left_index=True,
right_index=True, suffixes=('_homo', '_hetero'))
top50.hvplot.table(width=330)
We can also visualize our word frequencies. The classic way to do this is using a wordcloud. The package we use has a nice feature that allows you to define the outlines of your wordcloud. As an homage to Tinder we use this to make it look like a flame:
import matplotlib.pyplot as plt
mask = np.array(Image.open('./flame.png'))
wordcloud = WordCloud(
background_color='white', stopwords=stop, mask = mask,
max_words=60, max_font_size=60, scale=3, random_state=1
).generate(str(bio_text_homo + bio_text_hetero))
plt.figure(figsize=(7,7));
plt.imshow(wordcloud, interpolation='bilinear');
plt.axis("off")
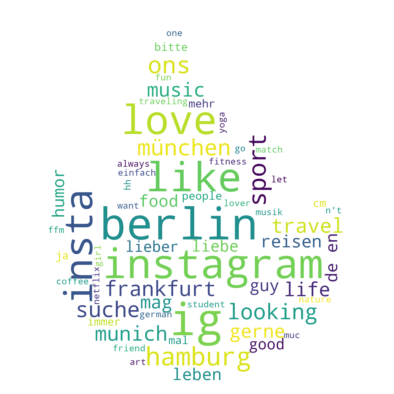
So, what do we see here? Well, people like to show where they are from especially if that is Berlin or Hamburg. That’s why the cities we swiped in are very common. No big surprise here. More interesting, we find the words ig
and love
ranked high for both treatments. In addition, for females we get the word ons and respectively friends for males. What about the most popular hashtags?
# Get hashtags from uncleaned text
hashtags = []
bio_text_dirty = ' '.join(profiles['bio'].tolist()).replace('\n', ' ')
for word in bio_text_dirty.split(' '):
if word.startswith('#') and len(word) > 1:
hashtags.append(word)
num_hashtags = Counter(hashtags)
hashtags_top10 = pd.DataFrame(num_hashtags.most_common(50), columns=['hashtag', 'count'])
hashtags_top10.hvplot.table(width=330)
It seems that people like to get creative with their hashtags and use whatever they feel like. Because of that the tags are not very repetitive. The reason might be that hashtags don’t really serve a purpose on Tinder. But here, as well as in the words investigated above we see that English words are pretty common. So, before we further investigate frequent words we’ll look at the share of German vs. English profile texts:
# Detect language for all cases with enough text
from langdetect import detect, lang_detect_exception
def lang_det(text):
try:
lang = detect(text)
except lang_detect_exception.LangDetectException as e:
pass
else:
return lang
profiles['lang'] = profiles['bio'].map(lambda x: lang_det(x))
# Get a sample of all profiles with some text and guess language
#profiles_sample = profiles.loc[profiles['bio'].str.len() > 3, ['treatment', 'bio']]\
# .sample(frac=0.1, random_state=1)
#profiles_sample['lang'] = profiles_sample['bio']\
# .map(lambda x: TextBlob(x).detect_language())
(profiles.groupby("treatment")['lang'].value_counts(normalize=True)\
.rename('rel. freq.') * 100).hvplot.bar(y="rel. freq.", x="lang", groupby="treatment",
alpha=0.4, muted_alpha=0.1)\
.overlay().opts(width=700, height=350,
title="Profile text languages",
xlabel="Language", yformatter="%.0f%%")
Using langdetect is the quickest way to detect the possible language of a text. However, it is a rather simple method. In contrast, the detect_language
method of TextBlob
works using the Google Translate API and is more elaborate. The downside being, that it takes way longer and has a limit on the amount of text we can submit. Consequently, we only take a random sample (10%) of our data to work on. Also, we should keep in mind that it also just returns a best guess. Hence, the results might be inaccurate especially when the text sample is short.
In our sample we see that on female profiles English is used almost as often as German and for gay males English is even the dominant language. While we observe a lot of different languages besides that their share is minuscule.
Without context the most common words from above are not very meaningful. What should we conclude from the word ons (one night stand)? Is Tinder really used for hook-ups only? Or do women rather express that they are not looking for that at all? We’ll find out soon:
# Words sourounding word of interest
words_closeby = []
for sentence in TextBlob(bio_text_dirty).sentences:
for word in sentence.words:
if word in ['ons']:
word_pos = sentence.words.index(word)
try:
words_closeby.append((sentence.words[word_pos-1],
# sentence.words[word_pos],
sentence.words[word_pos+1]))
except IndexError as e:
pass
most_common_words = Counter(words_closeby).most_common(10)
most_common_words
[(('keine', 'oder'), 18),
(('an', 'oder'), 9),
(('no', 'i'), 8),
(('an', 'f'), 7),
(('keine', 'keine'), 5),
(('no', 'or'), 5),
(('keine', 'und'), 5),
(('no', '🚫'), 4),
(('no', 'no'), 4),
(('keine', 'auf'), 3)]
The picture is clear: in most cases the texts state that they are in fact not interested in one night stands (“keine” means no) . Good thing we checked the context before drawing any conclusions.
Finally, we want to take a look at the emojis used in bios. We have seen before that they seem to be popular as they can carry a lot of meaning with few characters. With the help of the emoji package we can easily identify them in texts:
# Extract all emojis and count occurences
import emoji
emojis_unicode_homo = []
emojis_unicode_hetero = []
for word in TextBlob(bio_text_homo).words:
if word in emoji.EMOJI_UNICODE.values():
emojis_unicode_homo.append(emoji.emojize(word))
for word in TextBlob(bio_text_hetero).words:
if word in emoji.EMOJI_UNICODE.values():
emojis_unicode_hetero.append(emoji.emojize(word))
emoji_homo = pd.DataFrame(Counter(emojis_unicode_homo).most_common(10), columns=['emoji','count'])
emoji_hetero = pd.DataFrame(Counter(emojis_unicode_hetero).most_common(10), columns=['emoji','count'])
emoji_homo.hvplot.bar(x='emoji', y='count', invert=True, flip_yaxis=True,
title='Top10 emojis males', xlabel='')\
.opts(fontsize={"yticks": 15, "title": 18}, width=300, height=350) +\
emoji_hetero.hvplot.bar(x='emoji', y='count', invert=True, flip_yaxis=True,
title='Top10 females', xlabel='')\
.opts(fontsize={"yticks": 15, "title": 18}, width=300, height=350)
Again, for both treatments it becomes clear that people often share their location. That’s why the pin emoji is regularly used. Moreover, the German flag is commonly used and for males there are quite a few more flags. For females, Smoking seems to be pretty unpopular while wine and dogs seem to be well liked. Also, photography (or linking ones instagram) seems to be popular.
Coming up next
This concludes our analysis of the Tinder profiles data in which we uncovered a bunch of interesting patterns. Most prominently, we have found stark differences between the profiles of heterosexual women and homosexual men.
In this follow up post, I dive deeper into the Tinder game: I’ll present the results of an unique Tinder field experiment. Analyzing swiping and matching patterns I’ll present novel findings regarding discrimination in online dating.